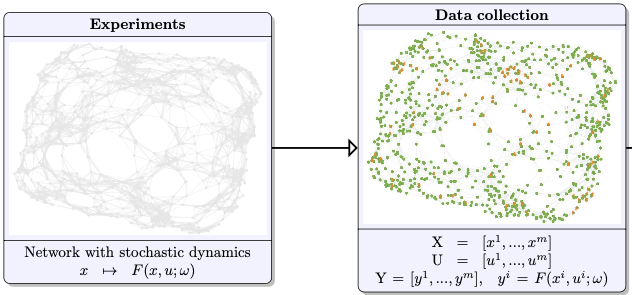
Networks are landmarks of many complex phenomena where interweaving interactions between different agents transform simple local rule-sets into nonlinear emergent behaviors. While some recent studies unveil associations between the network structure and the underlying dynamical process, identifying stochastic nonlinear dynamical processes continues to be an outstanding problem. Here we develop a simple data-driven framework based on operator-theoretic techniques to identify and control stochastic nonlinear dynamics taking place over large-scale networks. The proposed approach requires no prior knowledge of the network structure and identifies the underlying dynamics solely using a collection of two-step snapshots of the states. This data-driven system identification is achieved by using the Koopman operator to find a low dimensional representation of the dynamical patterns that evolve linearly. Further, we use the global linear Koopman model to solve critical control problems.
The human brain stores and processes new information from its environment and uses that information to adapt its behaviors. These complex processes of cognition and behavior can be conceptualized as emerging from more basic computational processes (information storage and compression, functional approximation) that are physically realized in the activity of a large number of interconnected neurons passing chemoelectric messages.
However, the causal link between neuronal behavior in a network to the emergent dynamic regularities is unknown. Neurons are subject to physical and biological constraints, limiting how they change their structure and connectivity in response to inputs. These restrictions hinder how physical networks of neurons can perform computational tasks. Artificial neural networks (ANNs), though inspired by neurobiology, are biologically implausible in structure or estimation method \cite{beerOneStepBack2019}. This limits the ability to draw inferences about the functioning of real neuronal networks.